Last weekend, Hollie Mengert woke up to an email pointing her to a Reddit thread, the first of several messages from friends and fans, informing the Los Angeles-based illustrator and character designer that she was now an AI model.
The day before, a Redditor named MysteryInc152 posted on the Stable Diffusion subreddit, “2D illustration Styles are scarce on Stable Diffusion, so I created a DreamBooth model inspired by Hollie Mengert’s work.”
Using 32 of her illustrations, MysteryInc152 fine-tuned Stable Diffusion to recreate Hollie Mengert’s style. He then released the checkpoint under an open license for anyone to use. The model uses her name as the identifier for prompts: “illustration of a princess in the forest, holliemengert artstyle,” for example.
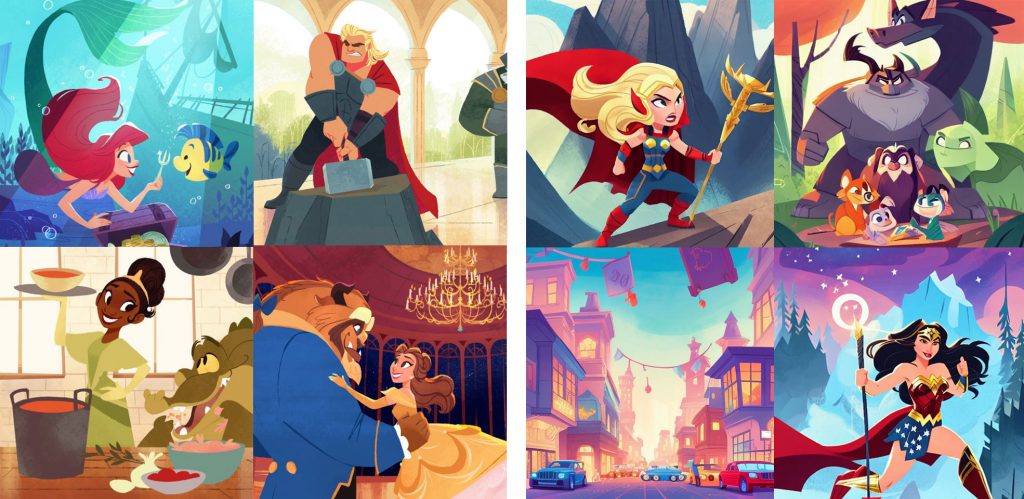
The post sparked a debate in the comments about the ethics of fine-tuning an AI on the work of a specific living artist, even as new fine-tuned models are posted daily. The most-upvoted comment asked, “Whether it’s legal or not, how do you think this artist feels now that thousands of people can now copy her style of works almost exactly?”
Great question! How did Hollie Mengert feel about her art being used in this way, and what did MysteryInc152 think about the explosive reaction to it? I spoke to both of them to find out — but first, I wanted to understand more about how DreamBooth is changing generative image AI.
Since its release in late August, I’ve written about the creative potential and complex ethical and legal debates unleashed by the open-source release of Stable Diffusion, explored the billions of images it was trained on, and talked about the data laundering that shields corporations like Stability AI from accountability.
By now, we’ve all heard stories of artists who have unwillingly found their work used to train generative AI models, the frustration of being turned into a popular prompt for people to mimic you, or how Stable Diffusion was being used to generate pornographic images of celebrities.
But since its release, Stable Diffusion could really only depict the artists, celebrities, and other notable people who were popular enough to be well-represented in the model training data. Simply put, a diffusion model can’t generate images with subjects and styles that it hasn’t seen very much.
When Stable Diffusion was first released, I tried to generate images of myself, but even though there are a bunch of photos of me online, there weren’t enough for the model to understand what I looked like.
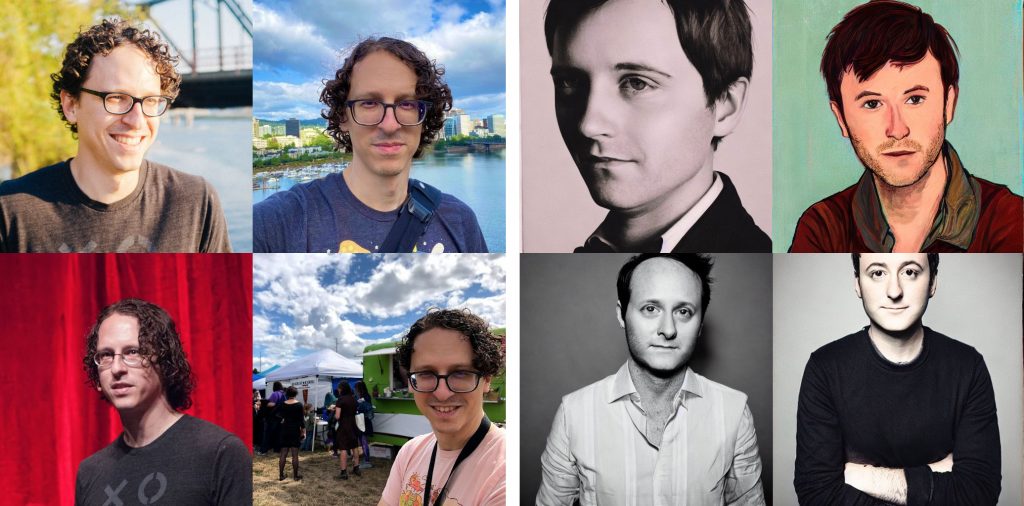
That’s true of even some famous actors and characters: while it can make a spot-on Mickey Mouse or Charlize Theron, it really struggles with Garfield and Danny DeVito. It knows that Garfield’s an orange cartoon cat and Danny DeVito’s general features and body shape, but not well enough to recognizably render either of them.
On August 26, Google AI announced DreamBooth, a technique for introducing new subjects to a pretrained text-to-image diffusion model, training it with as little as 3-5 images of a person, object, or style.
Google’s researchers didn’t release any code, citing the potential “societal impact” risk that “malicious parties might try to use such images to mislead viewers.”
Nonetheless, 11 days later, an AWS AI engineer released the first public implementation of DreamBooth using Stable Diffusion, open-source and available to everyone. Since then, there have been several dramatic optimizations in speed, usability, and memory requirements, making it extremely accessible to fine-tune it on multiple subjects quickly and easily.
Yesterday, I used a simple YouTube tutorial and a popular Google Colab notebook to fine-tune Stable Diffusion on 30 cropped 512×512 photos of me. The entire process, start to finish, took about 20 minutes and cost me about $0.40. (You can do it for free but it takes 2-3 times as long, so I paid for a faster Colab Pro GPU.)
The result felt like I opened a door to the multiverse, like remaking that scene from Everything Everywhere All at Once, but with me instead of Michelle Yeoh.
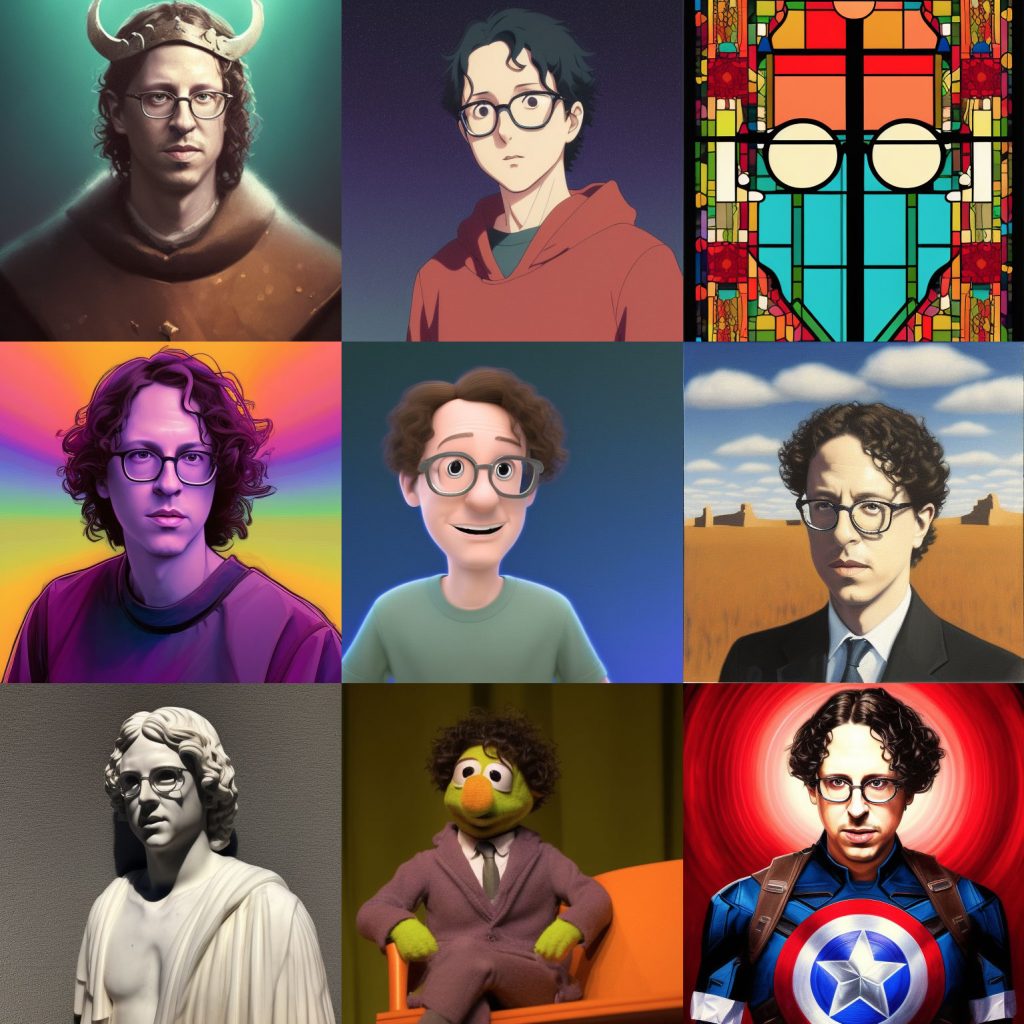
Frankly, it was shocking how little effort it took, how cheap it was, and how immediately fun the results were to play with. Unsurprisingly, a bunch of startups have popped up to make it even easier to DreamBooth yourself, including Astria, Avatar AI, and ProfilePicture.ai.
But, of course, there’s nothing stopping you from using DreamBooth on someone, or something, else.
I talked to Hollie Mengert about her experience last week. “My initial reaction was that it felt invasive that my name was on this tool, I didn’t know anything about it and wasn’t asked about it,” she said. “If I had been asked if they could do this, I wouldn’t have said yes.”
She couldn’t have granted permission to use all the images, even if she wanted to. “I noticed a lot of images that were fed to the AI were things that I did for clients like Disney and Penguin Random House. They paid me to make those images for them and they now own those images. I never post those images without their permission, and nobody else should be able to use them without their permission either. So even if he had asked me and said, can I use these? I couldn’t have told him yes to those.”
She had concerns that the fine-tuned model was associated with her name, in part because it didn’t really represent what makes her work unique.
“What I pride myself on as an artist are authentic expressions, appealing design, and relatable characters. And I feel like that is something that I see AI, in general, struggle with most of all,” Hollie said.
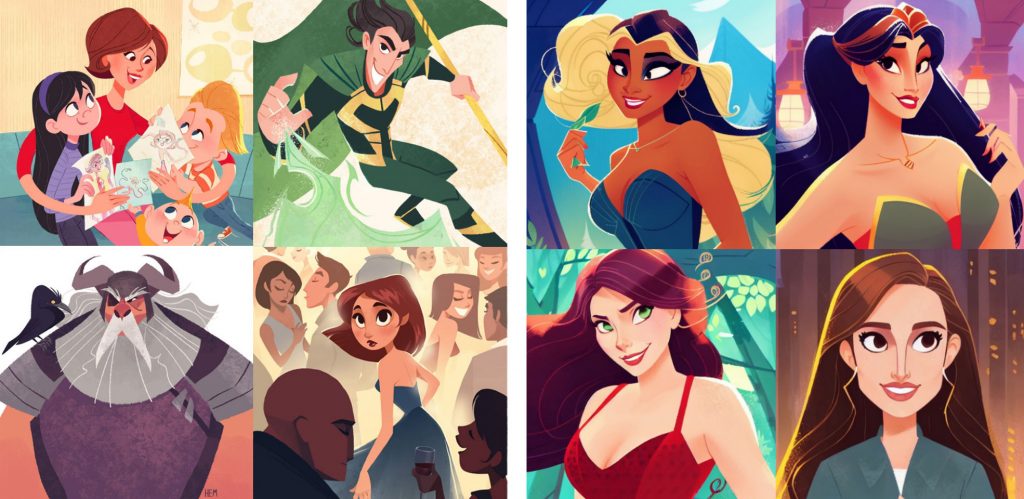
“I feel like AI can kind of mimic brush textures and rendering, and pick up on some colors and shapes, but that’s not necessarily what makes you really hireable as an illustrator or designer. If you think about it, the rendering, brushstrokes, and colors are the most surface-level area of art. I think what people will ultimately connect to in art is a lovable, relatable character. And I’m seeing AI struggling with that.”
“As far as the characters, I didn’t see myself in it. I didn’t personally see the AI making decisions that that I would make, so I did feel distance from the results. Some of that frustrated me because it feels like it isn’t actually mimicking my style, and yet my name is still part of the tool.”
She wondered if the model’s creator simply didn’t think of her as a person. “I kind of feel like when they created the tool, they were thinking of me as more of a brand or something, rather than a person who worked on their art and tried to hone things, and that certain things that I illustrate are a reflection of my life and experiences that I’ve had. Because I don’t think if a person was thinking about it that way that they would have done it. I think it’s much easier to just convince yourself that you’re training it to be like an art style, but there’s like a person behind that art style.”
“For me, personally, it feels like someone’s taking work that I’ve done, you know, things that I’ve learned — I’ve been a working artist since I graduated art school in 2011 — and is using it to create art that that I didn’t consent to and didn’t give permission for,” she said. “I think the biggest thing for me is just that my name is attached to it. Because it’s one thing to be like, this is a stylized image creator. Then if people make something weird with it, something that doesn’t look like me, then I have some distance from it. But to have my name on it is ultimately very uncomfortable and invasive for me.”
I reached out to MysteryInc152 on Reddit to see if they’d be willing to talk about their work, and we set up a call.
MysteryInc152 is Ogbogu Kalu, a Nigerian mechanical engineering student in New Brunswick, Canada. Ogbogu is a fan of fantasy novels and football, comics and animation, and now, generative AI.
His initial hope was to make a series of comic books, but knew that doing it on his own would take years, even if he had the writing and drawing skills. When he first discovered Midjourney, he got excited and realized that it could work well for his project, and then Stable Diffusion dropped.
Unlike Midjourney, Stable Diffusion was entirely free, open-source, and supported powerful creative tools like img2img, inpainting, and outpainting. It was nearly perfect, but achieving a consistent 2D comic book style was still a struggle. He first tried hypernetwork style training, without much success, but DreamBooth finally gave him the results he was looking for.
Before publishing his model, Ogbogu wasn’t familiar with Hollie Mengert’s work at all. He was helping another Stable Diffusion user on Reddit who was struggling to fine-tune a model on Hollie’s work and getting lackluster results. He refined the image training set, got to work, and published the results the following day. He told me the training process took about 2.5 hours on a GPU at Vast.ai, and cost less than $2.
Reading the Reddit thread, his stance on the ethics seemed to border on fatalism: the technology is inevitable, everyone using it is equally culpable, and any moral line is completely arbitrary. In the Reddit thread, he debated with those pointing out a difference between using Stable Diffusion as-is and fine-tuning an AI on a single living artist:
There is no argument based on morality. That’s just an arbitrary line drawn on the sand. I don’t really care if you think this is right or wrong. You either use Stable Diffusion and contribute to the destruction of the current industry or you don’t. People who think they can use [Stable Diffusion] but are the ‘good guys’ because of some funny imaginary line they’ve drawn are deceiving themselves. There is no functional difference.
On our call, I asked him what he thought about the debate. His take was very practical: he thinks it’s legal to train and use, likely to be determined fair use in court, and you can’t copyright a style. Even though you can recreate subjects and styles with high fidelity, the original images themselves aren’t stored in the Stable Diffusion model, with over 100 terabytes of images used to create a tiny 4 GB model. He also thinks it’s inevitable: Adobe is adding generative AI tools to Photoshop, Microsoft is adding an image generator to their design suite. “The technology is here, like we’ve seen countless times throughout history.”
Toward the end of our conversation, I asked, “If it’s fair use, it doesn’t really matter in the eye of the law what the artist thinks. But do you think, having done this yourself and released a model, if they don’t find flattering, should the artist have any say in how their work is used?”
He paused for a few seconds. “Yeah, that’s… that’s a different… I guess it all depends. This case is rather different in the sense that it directly uses the work of the artists themselves to replace them.” Ultimately, he thinks many of the objections to it are a misunderstanding of how it works: it’s not a form of collage, it’s creating new images and clearly transformative, more like “trying to recall a vivid memory from your past.”
“I personally think it’s transformative,” he concluded. “If it is, then I guess artists won’t really have a say in how these models get written or not.”
As I was playing around with the model trained on myself, I started thinking about how cheap and easy it was to make. In the short term, we’re going to see fine-tuned for anything you can imagine: there are over 700 models in the Concepts Library on HuggingFace so far, and trending in the last week alone on Reddit, models based on classic Disney animated films, modern Disney animated films, Tron: Legacy, Cyberpunk: Edgerunners, K-pop singers, and Kurzgesagt videos.
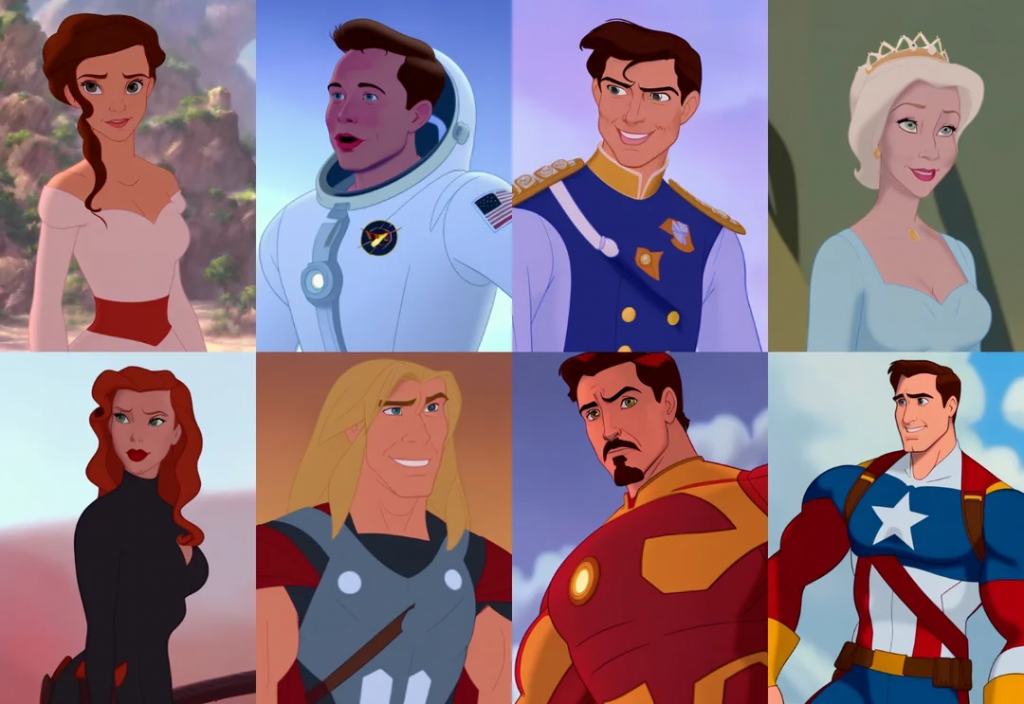
Aside from the IP issues, it’s absolutely going to be used by bad actors: models fine-tuned on images of exes, co-workers, and, of course, popular targets of online harassment campaigns. Combining those with any of the emerging NSFW models trained on large corpuses of porn is a disturbing inevitability.
DreamBooth, like most generative AI, has incredible creative potential, as well as incredible potential for harm. Missing in most of these conversations is any discussion of consent.
The day after we spoke, Ogbogu Kalu reached out to me through Reddit to see how things went with Hollie. I said she wasn’t happy about it, that it felt invasive and she had concerns about it being associated with her name. If asked for permission, she would have said no, but she also didn’t own the rights to several of the images and couldn’t have given permission even if she wanted to.
“I figured. That’s fair enough,” he responded. “I did think about using her name as a token or not, but I figured since it was a single artist, that would be best. Didn’t want it to seem like I was training on an artist and obscuring their influence, if that makes sense. Can’t change that now unfortunately but I can make it clear she’s not involved.”
Two minutes later, he renamed the Huggingface model from hollie-mengert-artstyle
to the more generic Illustration-Diffusion
, and added a line to the README, “Hollie is not affiliated with this.”
Two days later, he released a new model trained on 40 images by concept and comic book artist James Daly III.
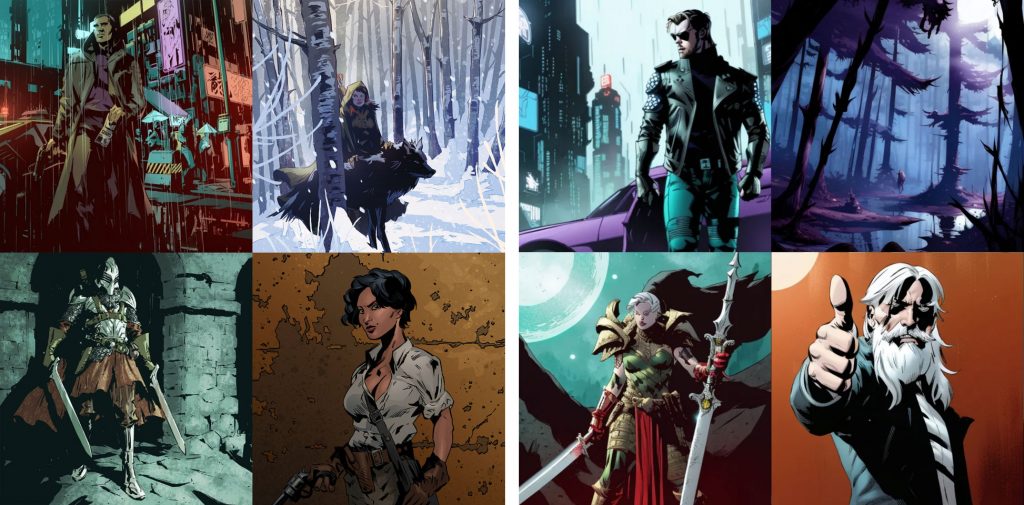